A new study calculates that twenty percent of all time “lost” in travel is due to traffic lights
Finally, proof for the Lachner Theorem:Â Traffic signals are a major cause of traffic delay
Another classic example of pseudo-science:Â Big data and bad assumptions produce meaningless results
When I was in graduate school, I shared a house in Berkeley with five roommates. Once a week we’d pool our food dollars, and pile into Archie Lachner’s ’67 Falcon and drive across town to Lucky, Safeway or the Co-Op, and mount a group shopping expedition for the week. This was in the late 70s, just after Berkeley had installed a series of traffic diverters to stop cut-through driving in residential neighborhoods. Our driver, Archie, repeatedly chose routes that were blocked by one diverter and then another. He cursed at the inconvenience: “These traffic diverters, they get in your way, they slow you down.” That prompted a heated debate about the merits of diverters. Archie defended the inherent right of drivers to go wherever they wanted. Others in the car said they could see how people who lived on these streets might appreciate the diverters cutting down on or at least slowing traffic. Archie had to turn around at least twice to avoid diverters, and as we finally got near the grocery store, we came to to a stop at a red traffic signal. From the back seat, someone said: “These traffic lights, they get in your way, they slow you down.”  Offended, Archie, spun the wheel and drove home–“if you can’t respect the driver, you won’t get a ride.” Despite the protests, Archie drove a couple of miles back home, and the five other roommates had to repeat the trip in another car.
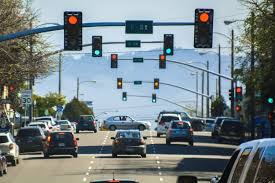
Thus was born the Lachner theory of traffic congestion: Traffic lights get in your way and slow you down. For decades the theory has been wanting for actual quantification, but at last, we have it. Crack statisticians at the University of Maryland have sifted through reams, nay gigabytes, of big data, and have produced a comprehensive, nationwide estimate of the amount of time lost when we sit, waiting for red lights to turn green.
According to these University of Maryland estimates, time lost sitting at traffic signals amounts to 329 million vehicle hours of delay, and costs us $8.6 billion dollars per year. Time spent waiting at traffic signals is roughly three-fifths as great as the 561 million vehicle hours of delay associated with routine “recurring” traffic congestion.
This new study from the University of Maryland finally vindicates the Lachner theorem. By their reckoning, roughly 19 percent of all traffic congestion is due to waiting at traffic signals. Those traffic lights do get in your way and slow you down.
Traffic signals cause delays as vehicles queue at intersections. In 10 states, traffic signals are the top cause of traffic congestion, though congestion levels overall remain relatively low in those states. For example, even though Alaska ranked highest in the country in percentage of delay caused by Signals at 53%, it ranked 42nd in terms of total hours of delay caused by signals.
As an accounting exercise, there’s little reason to doubt these calculations. But whether they constitute a “loss” is highly doubtful, because there’s no question that we’d all collectively lose more time in travel if there were no traffic lights. The policy implication of this finding is not that we should be tearing out or turning off traffic signals. That would be absurd, of course. And what the claims of time spent waiting at traffic lights constitute an actual “loss” rests on the assumption that there’s some other traffic-light free way of managing the flow of traffic at intersections that would involve less total travel time for those now waiting. Simply getting rid of traffic lights—and say replacing them with stop signs—would likely decrease the throughput of many intersections and actually increase delays (though it might beneficially reduce traffic speeds and improve safety for vulnerable road users). Theoretically one might replace every single traffic light in the US with a fully grade separated interchange without stops.
Let’s suppose, for a moment, that you could instantly replace all of the 330,000 or so traffic signals in the US with grade-separated interchanges that eliminated traffic signals. That might eliminate all the time “lost” by vehicles waiting at traffic lights, but it would come at a cost. At say, $10 million per intersection (which is probably a conservative estimate) that would cost about $3.3 trillion, all that to save maybe $8.6 billion per year. Time spent waiting at traffic lights is costly, only if you ignore the vastly greater cost of doing anything to try to reduce it.
It’s easy to point out that the Lachner Theorem about the “time loss” due to traffic lights is pretty silly. But what’s true of the elaborate (but fundamentally wrong-headed) estimates of the time “lost” to traffic signals is that it also holds for all the other estimates of supposed congestion costs. For years, a range of highly numerate charlatans have been purporting to compute the value of time lost to traffic congestion. The congestion cost studies generated by the Texas Transportation Institute, Inrix, Tom-Tom and others invariably conclude that traffic congestion costs us billions of dollars a year. Their copious data creates the illusion of statistical precision without providing any actually useful knowledge. They generate heat, but don’t shed any light: The congestion cost estimates are part of the propaganda effort of the road-builders, who assert we need to spend even more billions to widen roads to recoup these losses.
It’s an example of a measurement that’s literally true, but quite meaningless. It’s true in the sense that people probably due spend millions of hours, collectively sitting at traffic lights or traveling more slowly because of congestion. It’s meaningless, because there’s not some real world alternative where you could build enough road capacity to eliminate these delays. So, as an elaborate accounting exercise, you can use big data and computing power to produce this estimate, but the result is a factoid that conveys no useful, actionable information—just as we’ve shown with our Cappuccino Congestion Index, which totes up the billions of dollars American’s “lose” waiting in line at coffee shops.
The sky’s the limit if you want to generate large estimates of the supposed time “lost” to slower than imaginable travel. Consider for example flying cars or helicopters. If you could travel by helicopter to all your destinations, it would shave hours a day off your total travel time. With a spreadsheet and some travel data, you could work out an estimate of how many million hours might be saved and how many billions of dollars that saved travel time would be worth. You could produce a report arguing that the personal helicopter shortage costs us in lost time and money. It would be a large but meaningless number, because there’s no world where its financially feasible, much less physically possible, for everyone to take every trip by helicopter.
The only way to make meaning of such numbers is in the context of plausible, real-world alternatives. And that’s exactly what these cost of congestion studies almost invariably fail to consider. Something is only a “cost” if there’s an actual practical alternative that would save the lost time without incurring even greater monetary costs in doing so. Imaginary savings from an impossible, or impossibly expensive alternative aren’t savings at all. All of the evidence about induced travel shows that expanding capacity to try and reduce time “lost” to congestion is ultimately futile: more capacity encourages more travel, induces more sprawl, and does nothing to reduce congestion and delay.
It’s a welcome sign that one recent report acknowledged this fundamental fact.  To their credit, at least Tom-Tom acknowledges that adding capacity is futile, or even-counterproductive:
Developing road infrastructures and increasing the capacity isn’t the solution. “When a new road is built, it is only a matter of time before more vehicles are added to the road, offsetting this initial easing: it’s called the traffic demand dilemma”, Ralf-Peter Schäfer said. Change behaviours and traffic patterns can make a significant difference. Congestion is non-linear: once traffic goes beyond a certain threshold, congestion increases exponentially. Discouraging drivers to drive during peak rush hour can lead to big improvements, as proven during the pandemic.
And the purveyors of congestion cost estimates almost never point to the only solution that’s been proven to reduce congestion: road pricing. Even a modest system of time-based user fees could dramatically reduce congestion.
It’s tempting to believe that more data will make the answers to our vexing problems, like traffic congestion, clearer. But the reverse is often true: an avalanche of big data obscure a fundamental truth. That’s what’s going on here.